ARTICLE
The age of AI for insights.
The unbridled evolution of generative AI is disrupting the world as we know it. What insights professionals should know when it comes to implications for the future of our practice.
by Asha Parmar, Associate Director, C Space
Arms race. Revolution. Gold rush.
Call it what you like; the unbridled evolution of generative AI we see disrupting the tech landscape heralds a moment in history for global society and business at large.
We face a future that is both thrilling and riddled with complexity. Across sectors and functions, we share many questions:
What is it capable of?
How do we ensure it is trustworthy and relevant to our organization?
How do we stop it from becoming overwhelming?
To consider these questions and better understand AI and the future of insights, we ran a two-part series for The Better Why Peer Connections Sessions where we brought 45 insights leaders across categories together to discuss, learn from and inspire one another.
Keep reading for key learnings and questions we uncovered as a group, plus our next steps as insights professionals when it comes to navigating generative AI in the future world of research.
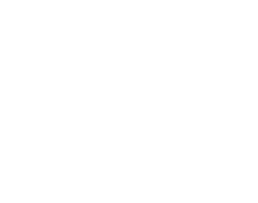
For generative AI in research, sheer scale is both its boon and peril.
Asha Parmar, Associate Director, C Space
AI isn’t goodbye to the researcher.
I liken the advent of more broadly accessible generative AI to a bicycle. It is a tool we have harnessed to move forward through the collaboration of man and machine. Without bikes, humans move more slowly. Without humans, bikes go nowhere.
Tech of this kind demands dialogue and collaboration to make meaningful progress. This, in turn, can help us build, nurture and organize around better relationships with our craft, culture and customers.
It’s
Relationship Thinking, and it’s
proven to drive better business.
Generative AI will not kill the researcher. I see our roles evolving to become more strategic than executional, demanding vision and the orchestration of tools.
Emphasis shifts from ‘getting it done’ to ‘how to get it done:’ setting up strategically rigorous sandboxes, defining prompts with precision, and coordinating powerful tools. It’s already clear that generative AI can be a collaborative thought partner and effective tool within the end-to-end research process; for concept creation, methodology design and moderation, insight synthesis, conversational analysis, storytelling, and data visualization to name a few.
In fact, Generative AI will be a force multiplier in research and training. It inspires as a sparring partner in brainstorms, offering thought starters, builds, and challenges. It drives velocity, synthesizing and charting huge swathes of data at an unmatched pace. It tackles the tedious, making room for higher-level thinking across teams and enriching our value-add.
But, for now, at least, these systems are goal-oriented and built to optimize. They struggle to adapt to new environments and if rules change — and they inevitably do as values shift and culture moves on.
We must continue to assess how these models match up to human expectations of particular goals. Misalignment occurs when goals are inadequately defined by humans, creating ambiguity in a desired outcome as well as through technical limitations to do with how the system was trained.
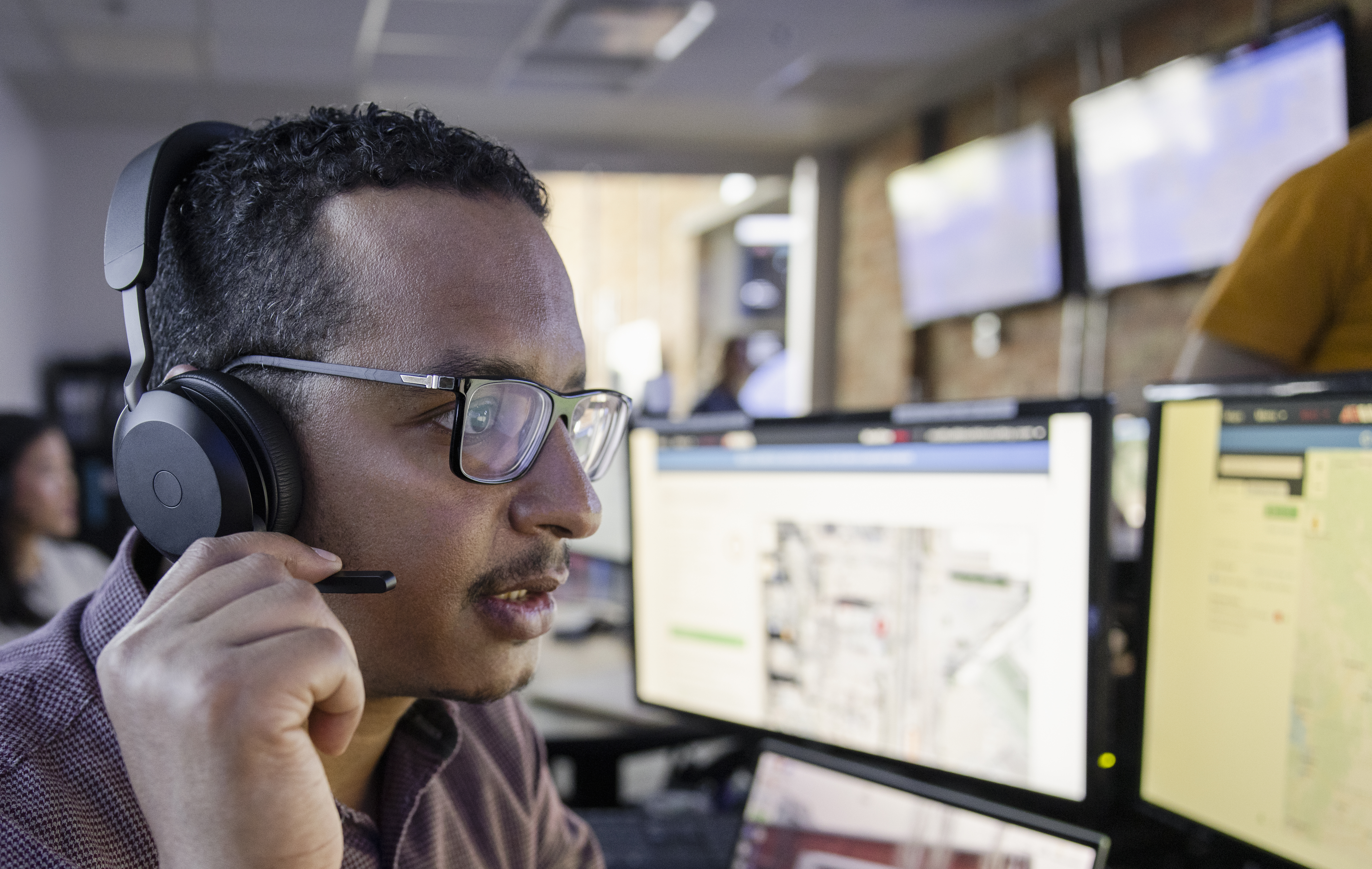
A threat to diversity and originality.
Models work by using deep neural networks to generate content based on patterns learned in training data. They are only as good (and diverse) as the people programming them and the data feeding them.
And let’s face it, we still live in a W.E.I.R.D. (Western, Educated, Industrialized, Rich, Democratic) world. Generative AI stands to perpetuate and amplify the inherent biases of today’s data and the society it reflects.
Applied critically, it may help us identify blindspots by flagging assumptions and intrinsic bias that we hold as humans, conditioned by our own lived experience. Sheer scale is both its boon and peril.
This raises critical questions for DEI. Though models are increasingly multi-lingual, limitations with training data mean for now they perform best in ‘proper’ English. Do we face a shift towards Anglocentrism and the erasure of cultural vernacular? Digital representation of languages is a key barrier to truly representative multilinguality. Indeed, how does a model decide what is valuable or interesting within the data? Whose voices in research are being prioritized over others?
When AI systems are deployed by different decision-makers across contexts but share a foundation model and central training data sources, they learn and predict from the same existing patterns. This risks a trend toward homogenized outcomes and algorithmic monoculture* which threatens to narrow the worldview and human truths they’re able to convey.
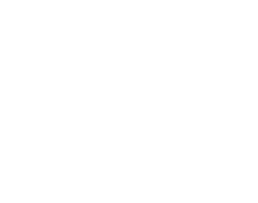
You have to control the use cases knowing there are downsides. Be prepared for biases. You need to build into your process how to control against it.
VP, Customer Experience Strategy, Financial Services Company
Keeping it real in an increasingly machine-led world.
To that end, as businesses focus on the commercial benefits of AI, they can lose sight of what people value. Philosopher Atoosa Kasirzadeh wrote, “The promise that AI technologies will benefit all of humanity is empty so long as we lack a nuanced understanding of what humanity is supposed to be.”**
We’re in the business of unearthing human truths.
No doubt, the tech has gotten good. Digital twins clone target customer segments using billions of data points (surveys, CRM data, reviews) allowing researchers to predictively model feedback before launching to living, breathing humans. LLM-driven interviewers can autonomously moderate IDIs in-language where businesses lack speakers. Blending facial coding with transcript analysis might help us spot, at scale, divergence between what’s expressed implicitly and explicitly: crooked eyebrows may belie a claim that all is well…
This kind of technology could allow us to bridge empathy and reach in ways never before possible through more robust qual/quant blends. But, how human can this truly be?
Machines cannot replicate lived experience. Analysis divorced from nuanced cultural, social and political context does a disservice to the customers we represent and the complexities of human truths. It cannot identify the negative space and read between the lines, triangulating what is there with what is not; what customers won’t say, can’t say, or don’t know.
Models only know what they’ve seen in the data, which, for now, is primarily written text. A wealth of cultural information and signals exists in non-written form, especially for societies whose primary form of cultural transmission is historically oral.
The ‘facts’ AI asserts could flatten the richness of the voices we seek to elevate.
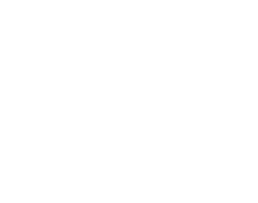
I don’t see AI replacing research. If anything, it makes qual have a little more value in my book. It’s not a replacement tool.
Product Marketing Insights Professional, Global Technology Giant
Remaining curious and critical is key.
Culture changes constantly. The nuanced interplay of imitation (what was) and lived experience (what I know to be) drives inspiration (what could be) and our capacity to innovate. If AI is fundamentally backward-looking and predictive, without lived experience to integrate, its potential to fuel true innovation remains stunted.
Opposing forces abound in this new dawn with light and dark sides at every turn: trust and suspicion, intuition and assumptions, intimacy and distance, support and demands.
Many of these tensions are familiar in individual and collective human:human relationships; and we have made our peace to some extent. In the context of machines, they ignite us anew.
Collaboration and dialogue with and about AI can help us elevate relationships with our craft, culture, and customers. Remaining curious and critical is paramount to successfully harnessing this tool.
Building the scale and velocity it offers into the community model can supercharge the agility, depth, and creativity of empathy-led research.
Replay a LinkedIn Live discussion with Citi and Xero to dive in further on the implications of generative AI for insights across categories, and how we are approaching AI at C Space.
So long as we remember how to use the gears, let’s enjoy the downhill ride.
*Bommasani, Rishi, et al. “Picking on the Same Person: Does Algorithmic Monoculture Lead to Outcome Homogenization?” ArXiv.org, 25 Nov. 2022, arxiv.org/abs/2211.13972. Accessed 6 June 2023.
**Kasirzadeh, Atoosa. “ChatGPT, Large Language Technologies, and the Bumpy Road of Benefiting Humanity.” ArXiv.org, 21 Apr. 2023, arxiv.org/abs/2304.11163. Accessed 6 June 2023.